A New Era of Travel: AI Chatbot Tips You Need to Know
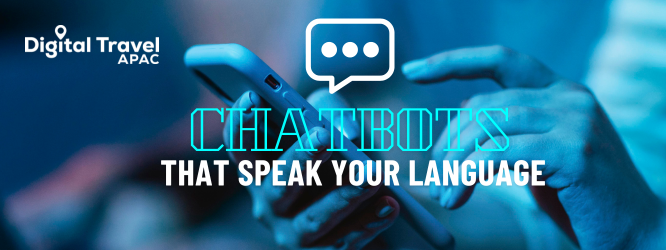
AI chatbots have become indispensable tools for businesses, offering 24/7 customer support, task automation, and enhanced customer experiences.
Our report Navigating Tomorrow: Retail Benchmarking with AI Insights reveals that 70% of customers engage with chatbots for product sales inquiries, highlighting their growing importance in the retail industry.
In a globalised world, businesses often face the challenge of language barriers, communicating effectively with customers from different regions who speak various dialects and languages.
This post-event exclusive Q&A interview with Preeti, VP Operations, from Go City APAC, explores how to navigate the complexities of guiding AI chatbots with pre-trained multilingual models, refine data processing for personalised itineraries and conducting sentiment analysis for more precise marketing strategies.
How AI Chatbots Can Adapt to Dialects
How can AI chatbots effectively interact with users speaking specific dialects of a language?
Chatbots can be trained on large datasets containing texts from different dialects of a specific language, including regional idioms and local expressions.
Depending on their targeted segments, AI chatbots can be trained to understand and respond to various dialects
Multilingual models can be incorporated to understand variations within a language. By fine-tuning these model on region-specific language variations, chatbots can adapt to local dialects
Case Study: Go City's Chatbot
What AI tools or products does Go City utilize to build its chatbot?
Go City works with 3P agencies and leverages their generative AI solutions, primarily built using OpenAI and ChatGPT.
How do you measure the effectiveness of your chatbot’s interactions with customers?
Each customer interaction is recorded and analysed using a combination of machine learning (intent recognition) and AI (sentiment analysis, deep conversational analytics).
Their effectiveness is measured on 2 levels: message level and case level.
At message level, every customer prompt is matched to an intent which forms the basis of the bot's reply. A bot will only reply if it can recognise the intent with 95% confidence. If the confidence level is below the threshold, the bot will ask for further clarification with prompts.
At case level, we measure containment, which indicates whether the bot was able to handle the case without needing to transfer it to a human agent. We also ask the customer to rate their customer support experience at the end of the interaction.
Key Features and Benefits
Can you explain how the trip planning feature works within Go City’s app?
Go City APAC has a trip planning feature on their App that helps travellers pick their attractions of interest and specify their travel dates to craft out an itinerary most suitable for the customer. This feature uses both Machine learning, AI and historical data to come up with the most personalised and recommended itineraries.
The company plans to integrate this feature into their bots in the future.
Is it possible to monitor social media sentiment across all platforms?
Social media monitoring across platforms for sentiment analysis is possible using Generative AI. However, the effectiveness depends on several factors, including platform-specific APIs, data accessibility, and the scope of monitoring required.
Go City has implemented social listening across popular channels like Facebook, Instagram, TikTok and more. Our 3rd party provider has standard integrations with these channels and many more.
With this they can monitor their brand presence to proactively pick up common themes, pain points and customer sentiments. In addition to this, they can determine marketing campaign effectiveness/reach and identify their biggest influencers - both promoters and detractors.
Challenges and Future Directions
Can the same technology stack be used across different countries, such as in China?
Go City works with partners who offer omnichannel support and have integrated with platforms like WeChat in China. The company builds a bot and then integrates that into their WeChat Mini program for China, making the same bot available to different platforms including Facebook, LiveChat, WeChat etc. They also use large language translated datasets for references and train the bot to offer customer support across different languages.
How does the chatbot handle conversations where customers mix languages, like Taglish?
The bot has been designed to be customer-centric, allowing customers to select any supported language or simply start chatting in their preferred language
If a customer mixes supported and non-supported languages, the bot will reply in the supported language and ask for clarification.
Our Contributor:
Preeti , VP Operations, APAC, Go City